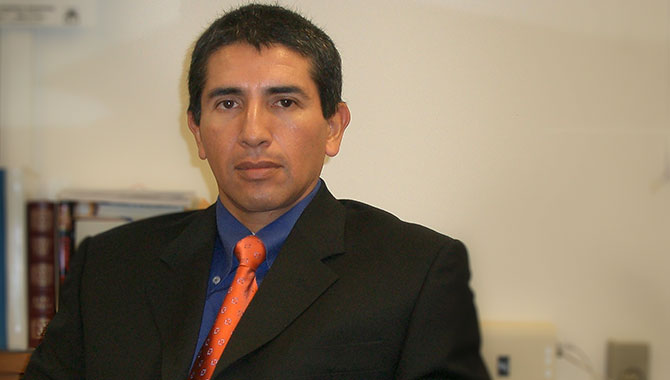
NASA ARC CKO Donald Mendoza.
Photo Credit: NASA
Donald Mendoza discusses knowledge sharing at NASA’s Ames Research Center.
Donald Mendoza is the Chief Knowledge Officer (CKO) for NASA’s Ames Research Center and chairman of the center’s Lessons Learned committee. Mendoza, a senior systems engineer, is responsible for establishing the policies and procedures that the center uses to capture and disseminate lessons learned. He has authored over 400 lessons learned covering a broad range of areas where he has experience and expertise, including flight tests, wind tunnel tests, applied research in fluid mechanics, photochemistry, photonics, air transportation, spacecraft technology, information technology, system safety and quality assurance. He performed his undergraduate and doctorate work at the California Polytechnic State University and the University of California, Berkeley, respectively.
You’ve been very successful in the area of lessons learned. What’s your view of the importance of capturing and organizing lessons learned?
It’s critical because many things that we do are not new, but a lot of the new things we do are based on what we did before. If this particular function isn’t exercised then people keep repeating not only the same successes, but they repeat the same mishaps, failures and missteps.
What does it take to make people aware of lessons that have been learned and give them an opportunity to apply lessons learned on other projects?
That’s always a big challenge. And I would say that for the most part the majority of what happens is very, very organic. What I mean by that is it’s all people-based. If a particular engineer or scientist or manager has had a particular success or failure in one area and they go to another, they usually take those experiences with them and the people around them benefit. So it’s very organic, and our job is to formalize that so it’s not so people-dependent. But unfortunately I haven’t found a true way to uncouple that. So the big challenge is convincing people that outside of the organic process, the artificial process can work.
What usually takes place here at Ames is a combination where if one particular individual has certain experience that has the potential to benefit someone else, we will write that up as concisely as possible and distribute it to the people that we think would benefit from that. And then on top of that we try to have the original source of the knowledge and they basically share their experience in a story or chat. That’s what has the most impact.
It’s a very difficult thing to basically refer someone to a giant database and say, ‘Here, go and queue the database for lessons on propulsion systems,’ or ‘Here’s a spreadsheet that has all the lessons from Mission A. See if any of them are applicable to Mission B.’ The success of that approach is not very good, and that’s why you always have to have some type of a human element involved. We try to engage the human element as much as possible, but that’s not always possible because oftentimes the human is no longer at the agency, so then we turn to the machinery of the process.
What are some of the tools and methods you’ve applied to be successful?
My take from what I’ve seen – when the human element isn’t there — is that the machinery needs to be almost transparent. I define “machinery” in this context as any digital database, including an Excel spreadsheet or Word document, that’s used to capture the words of a knowledge event. The machinery needs to be in the background. It needs to be secondary. Even with these audits like GAO and OMB, they come in and all they look at is the machinery. Based on that, they write up their criticisms of the agency and they’re sometimes unfounded. So that’s a good case of where the machinery actually works against you. It illustrates how people get lost in the machinery.
At JPL they have a program whereby once a lesson gets processed through their machinery, there’s a group of humans who then assess the knowledge or the output of the machinery and decide how to fold that into their business as usual. So what you’ll see is that at JPL everybody knows what the design principles are. The documentation guides how they do their work. And what are their successes based on? They’re based on people practicing what’s in these documents. When they get a lesson, they will assess it for which document needs to be enhanced or revised or changed based on that lesson. So rather than having the end user have to go to some specific database to look for a lesson on some part, they’re just doing their work. The knowledge is already embedded in the instructions that they’re using. That, I believe, is a fantastic way to manage corporate knowledge — to blend it into the standard way of doing business.
Are there any successful knowledge efforts in your organization that you’d like to highlight?
I’m going to go back to the machinery. So far I’ve been talking about the back end of the machinery and how difficult it is to get to that point. It’s equally difficult to get people to the front of the machine, which means getting people to put stuff in the machine. They don’t see the value of it as opposed to them going into a new work environment or a new project and just applying what they’ve learned and having other people pick up on that. As much as I criticize the machinery, it’s much needed and critical because people come and people go and that human element is tenuous, whereas the machine is just always there.
We use a hybrid method here at Ames that kind of blends the people and the machinery. For instance, people are working missions. Their time is very limited and, unfortunately, that means sometimes they don’t have time to stop and document their lessons. So we have an agent — a human — that facilitates that process. The way the process works is you have this agent, who is critical. The agent has to be well versed in all the work the center does. They have to be positioned in the organization where they have access to all the work that’s being done and privy to all the issues and successes that are going on as well. Then that person can actually direct where a knowledge management activity should occur.
To give an example, if I go to a project status meeting and I hear there’s a particular issue or success or accomplishment, then I can follow up with the particular person on the project that’s the root of the issue or the accomplishment. I can sit down with them for 20 minutes, and when I’m finished I will have basically a draft of the lesson learned. So it takes all of the hassle off the person who would otherwise have to be at the front end of the machine. And all it requires of them is that they sit down and tell me a story. I write it up and all they need to do is review it for accuracy to see if I captured the intent of what they were sharing. Once that’s all been agreed upon, then I can directly share that with people around the center who would benefit, and then at the same time, I can go and deposit it in the big machine. That process has been very, very successful whenever I have the time to perform it. And that’s the issue. It’s a great process, but it needs some time and dedication.
What’s the biggest misunderstanding that people have about knowledge?
It’s that people think the machine can retain or represent 100 percent of the knowledge event’s accuracy, and I think people miss the fact that there’s a lot of context and so much other information the machinery cannot retain. It’s that piece that’s connected to the human interaction — the intangible type of information. The biggest misconception is that the machine can do that. The next biggest, from an external standpoint, is that machines represent all there is to knowledge management. And if a database doesn’t get a certain amount of hits each month, then an organization must not be managing its knowledge.