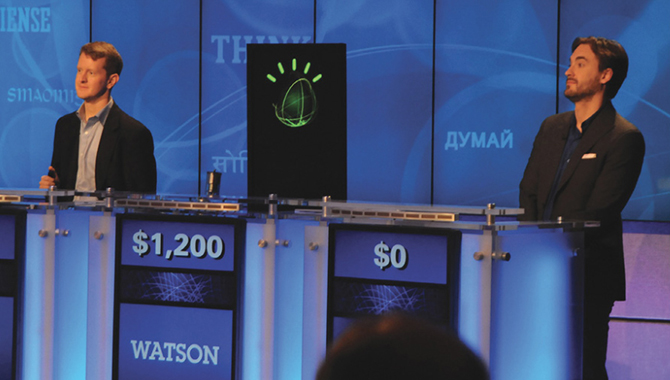
IBM’s Watson computer system competes against Jeopardy!’s two most successful and celebrated contestants—Ken Jennings and Brad Rutter.
Photo Credit: IBM
NASA’s need for the capacity to accumulate and make sense of vast amounts of material is what makes Watson a potentially valuable tool for NASA. The amount of technical content relevant to NASA missions and research projects is enormous and growing rapidly. The good news is that the information needed to solve most technical problems is almost certainly out there somewhere; the bad news is that the sheer volume of information can make finding and digesting the relevant content harder and more time consuming. That is why a number of us at the NASA Langley Research Center have been working with IBM on a pilot program to see if IBM’s Watson computer can help our researchers and engineers to analyze and digest the material they need as efficiently and effectively as possible and help them to develop innovative solutions.
Most people first became aware of IBM’s Watson computer when it outplayed human experts on the Jeopardy!® TV quiz show in 2011. The computer was able to answer questions posed in ordinary language that often demanded a knowledge of context and an ability to unravel complexities and ambiguities.
Watson understands questions, produces possible answers, analyzes evidence, computes confidence in its results, and delivers answers with associated evidence and confidence levels. It does this with a vast database of structured and unstructured content. Watson is used in a variety of fields, including medicine. It assists physicians, for instance, suggesting diagnoses and treatments.
Like the quiz-show Watson, the healthcare Watson compares symptoms and other information with its vast stores of content that includes medical literature, clinical trial results, and patient records to arrive at its recommended treatment paths with traceable evidence that a physician can use to make the best decision possible. The computer is not “smarter” than the doctors. A human-machine collaboration and symbiosis combines the computer’s mastery of vast quantities of relevant information—more than any human expert could absorb or remember—with the expertise, experience, and creative intelligence of the professionals who use it.
PILOT PROGRAM
We started to investigate the Watson technologies in 2011 and held a center-wide seminar on Watson that summer, including visiting Watson Labs in New York. In 2012, we chose to investigate the “content analytics” software part of the overall Watson suite for our proof of concept. We were comfortable choosing the content analytics element early, since we thought it would be the most helpful to address specific NASA information challenges for only a modest investment. Based on positive researchers’ response to the proof of concept, we formulated the “Knowledge Assistant” in 2013—our Langley-specific version of Watson Content Analytics (WCA) to search and analyze unstructured information from multiple sources and to quickly understand and deliver relevant insight with customizable facets and concept extraction. This pilot started in 2014 in collaboration with IBM WCA experts and Langley researchers/subject-matter experts.
We chose two technical areas—carbon nanotubes and autonomous flight—to test the WCA pilot concept. Both of these fields are technology incubator areas with a high potential for Center research and so are worth the investment of time and money.
Currently, subject matter experts (SMEs) in these and other fields have to read the entire literature corpus to digest and identify important information essentially “by hand.” Not only does the process take a lot of time, it is likely to miss valuable content and connections. We thought of the Watson knowledge assistant as a virtual helper to SMEs, one that could efficiently analyze the material and help them to readily find important connections between information as well as identifying experts in the field.
The first phase of our pilot, from January to August 2014, tested Watson Content Analytics’ ability to make sense of large bodies of knowledge, provide brief summaries of the key points of documents, and help SMEs discover potentially valuable trends, relationships, and experts. Reviewing content relevant to nanotube technology (approximately 130,000 documents), for instance, the machine generated automated clusters of documents on topics like the strength of reinforced composites and identified experts based on their contributions to the literature. These results were validated by SMEs.
The NASA SMEs involved in the pilot see the value of this kind of analytics—the ability to review all relevant materials and uncover connections that a human searcher would likely miss. The Knowledge Assistant had the ability to analyze the content in a variety of ways and identify important authors and experts on a range of technical subjects.
Phase 2 of the pilot, which began in September 2014 and was completed in February 2015, has focused especially on making the discovered content accessible and useful to researchers and engineers. This phase, working with IBM experts, has led to important progress on developing a more intuitive user interface, one that includes visualizations that make important information and connections apparent.
Watson Content Analytics uses syntactical and statistical sorting algorithms for clustering articles that share key terms, parses by “concepts,” and identifies top experts, possible collaborators, and linkages between concepts. For further knowledge extraction, these results have been augmented with semantic technologies such as taxonomies and custom concept rules, improvements that required collaboration with subject matter experts. Our pilot showed that close collaboration with content analytics experts and mission team members who need information is critical to success.
The Knowledge Assistant pilot with its improved user interface has been demonstrated widely to many SMEs and senior technical leaders; their feedback on its usefulness is very positive. One of the challenges we have to consider is the cost of purchasing the scholarly content the system needs access to for deep analytics. Buying all the scholarly content that might be relevant is prohibitively expensive. So our current plan is to offer WCA as a capability to analyze the content that is internal to NASA, open aerospace content including NASA reports, and the research collections of individual users.
THE FUTURE
The future potential of analytics technologies seems especially great. We are pursuing a full proof of concept to demonstrate the full functionality of these analytical capabilities that we believe will become an essential tool for researchers and engineers currently overwhelmed by the quantity and complexity of information in their fields.
We have just started to work with IBM Watson experts to develop an Aerospace Innovation Advisor Proof of Concept using IBM Watson Discovery Advisor technology, which is currently being used in medicine. Our aim is to demonstrate how natural language processing and machine learning technologies can be applied to aerospace research and development to accelerate the pace of discovery and innovation by analyzing and fully leveraging massive amounts of technical information. Watson Discovery Advisor technology is designed to boost analysis, provide valuable insights, and inspire research by finding connections, insights, and hidden relationship that human experts are unlikely to find. It can answer questions and even suggest questions that researchers have not thought to ask.
Looking further into the future, we can imagine a ”Virtual/Intelligent Agent” that is a true collaborator with an expert in a human-machine partnership. Such a system might be able to read relevant scientific literature in a variety of foreign languages, understand mathematical equations and tables, and relate it to material in English. It might understand multimedia content: images, figures, formulae, and videos. And, most important, it might be able to provide direct answers or lists of possible answers to users’ questions (rather than just lists of potentially useful documents). In those cases, as in its current use for medical diagnosis, the system would provide not just a set of possible answers but also information about the evidence it used to arrive at them, so the human experts will have the information they need to evaluate the conclusions. The system would be a true collaborator in our future research and engineering development efforts.
Note: Trade names and trademarks are used in this report for identification only. Their usage does not constitute an official endorsement, either expressed or implied, by the National Aeronautics and Space Administration.
Manjula Ambur is the Leader of Big Data Analytics and Chief Knowledge Officer at NASA Langley Research Center.
Don Cohen is Editor-in-Chief of NASA Knowledge Journal.